Day 1 - June 07
Poster Competition: Data-IQ: Characterize & Audit your training data with 2 lines of code!
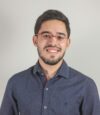
Nabeel Seedat
High model performance, on average, can hide that models may systematically underperform on subgroups of the data. To tackle this, we propose Data-IQ, a framework to systematically stratify examples into subgroups with respect to their outcomes --- allowing users to audit their tabular, image or text data with just two lines of extra code!
We do this by analyzing the behavior of individual examples during training, based on their predictive confidence and, importantly, the aleatoric (data) uncertainty. Capturing the aleatoric uncertainty permits a principled characterization and then subsequent stratification of data examples into three distinct subgroups (Easy, Ambiguous, Hard). We show that Data-IQ's characterization of examples is most robust to variation across similarly performant (yet different models), compared to baselines. Since Data-IQ can be used with any ML model (including neural networks, gradient boosting etc.), this property ensures consistency of data characterization, while allowing flexible model selection. Taking this a step further, we demonstrate that the subgroups enable us to construct new approaches to both feature acquisition and dataset selection. Furthermore, we highlight how the subgroups can inform reliable model usage, noting the significant impact of the Ambiguous subgroup on model generalization.