Day 1 - June 07
Generating Synthetic Tabular Data That’s Differentially Private
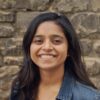
Lipika Ramaswamy
While generative models are able to produce synthetic datasets that preserve the statistical qualities of the training dataset without identifying any particular record in the training dataset, most generative models to date do not offer mathematical guarantees of privacy that can be used to facilitate information sharing or publishing. Without such mathematical guarantees, each adversarial attack on these models and the synthetic data they generate needs to be thwarted reactively. We can never be aware of adversarial attacks that might become feasible in the future. This is exactly the problem that differential privacy (DP) solves by bounding the probability that a compromising event occurs. By introducing calibrated noise into an algorithm, DP defends against all future privacy attacks with a high probability. In this session, we’ll explore approaches to applying differential privacy, including one that relies on measuring low dimensional distributions in a dataset combined with learning a graphical model representation. We'll end with a preview of Gretel's new generative model that applies this method to create high-quality synthetic tabular data that is differentially private.